Banks continue to look for ways to incorporate financial technology, or FinTech, into their daily operations in order to be more profitable and provide better services to their customers. Machine learning has the potential to assist banks in these areas. While Hollywood imagines machine learning as robots overthrowing humans, the reality of ML is much less dramatic, however, it does have the potential to revolutionize the banking industry through practical applications. Machine learning uses algorithms, rather than a set of explicitly programmed instructions, in order to analyze information, make decisions and learn from those decisions. Over time, ML then modifies its own code, without human oversight, so it can make better, faster, and more accurate decisions.
Cybersecurity
In the digital age, no one seems to be safe from cyberattacks. From huge corporations, such as Target, to government institutions, like the U.S. Department of Justice and the Internal Revenue Service, there seems to be no shortage of entities who’ve suffered cyberattacks. The financial industry is especially vulnerable to attacks due to the financial and sensitive customer information hackers have to gain. In order to protect customers and preserve trust, it’s vital for institutions to continue to look for ways to protect personal information.
Machine learning solutions, combined with technology in biometrics, are being developed to help protect sensitive customer information. Machine learning can be used to detect how much pressure a person uses to type, the speed at which they type, and the way they navigate a webpage. If an algorithm detects a change in a user’s typical behavior, the system will ask for the user to confirm their identity through facial or voice recognition. The power of machine learning and its ability to process vast amounts of data at a high speed also plays a role in cybersecurity. Machine learning can analyze and recognize suspicious patterns across company networks, flagging network administrators of potential hackers. It’s impossible for humans or traditional technology to process big data at the speed and accuracy of machine learning technology, giving companies a new, fighting defense over hackers.
Smart trading
The application of machine learning is highly suited for the financial industry due to reliable historical trends, high volume of data, and its quantitative nature. For years, automated functions have been used to buy and sell stock at certain prices but consumers are demanding more from technology to help them make smart trading decisions. As technology advances and machine learning has more practical applications, there’s little doubt ML will grow in the area of smart trading.
The power in machine learning technology comes from its ability to mine enormous amounts of data. The more data it processes, the more accurate its predictions. By leaving trading decisions up to machines, investors are able to remove human bias from the equation, helping make calculated, accurate decisions. Machine learning in trading also helps eliminate the cost of paying a financial advisor. A lower cost for financial advice opens up investing to new, less-affluent customers. While demand for automatic trading is expected to grow, it’s unlikely machines will replace humans. Customers are reluctant to put their entire trust into machines and expect human oversight of the decisions being made that ultimately affect them. The best possible solution is for machines and humans to work together in financial trade decisions.
Regulation
Companies face enormous operating costs in order to keep up with Know Your Customer (KYC), Anti-Money Laundering (AML) and Bank Secrecy Act (BSA) laws. Since 2007, the world’s top 50 banks have spent $173 billion on new staff and systems to make sure that they are compliant, according to financial services consultancy Quinlan & Associates. Not only are the risk management costs high but banks also face paying enormous fees for being out of compliance. While regulations are intended to lower banks’ risk, increase transparency and protect customers, keeping up with compliance can be a challenge.
While still early in its practical application, machine learning has great potential to help banks keep up with regulatory compliance. As machine learning technologies assume new regulations, they will have the ability to identify where it’s applicable and analyze gaps in current practices. This will help banks keep up with the constant flux in regulations with increased speed. Machine learning can also be taught how to identify suspicious activities with greater accuracy, reducing false red flags. Since financial regulations are expected to increase, machine learning could provide an invaluable solution to help banks keep up with compliance, reducing the cost and increasing its accuracy.
Fighting fraud
Detecting fraudulent activity quickly is crucial for any financial institution. Current systems lack the computing power and big data to identify accurate alerts of fraud. This results in wasted resources investigating legitimate transactions rather than real fraudulent activity. The faster banks are able to analyze and respond to unusual activity, such as out-of-state purchases or large cash withdrawals, the quicker they can take control of an account, minimizing any losses, better protecting their customers.
By analyzing the transactional history of an account against thousands of data points, machine learning can process deep data to determine the likelihood of fraud. Machine learning programs learn from each transaction and each decision the software makes, allowing the program to make speedier and more accurate decisions with each transaction. Over time, algorithms can even adjust themselves to changing financial habits of the account owner over their lifetime.
Chatbots
Even in the digital age, providing quality customer service remains a top priority for banks. Meeting the demand for speed and convenience without losing the human element is important to banks and their customers. Older automated phone support systems leave the conversation one-sided, as they run through the list of options, unable to solve unique customer issues. A problem with older chatbots is that they cannot recognize grammatical errors in human input, which results in a very rigid and unnatural response or no answer at all.
Machine learning has the potential to improve automated systems, such as chatbots, when it comes to customer service. Through proper training, machine learning systems can recognize patterns, emotions, and natural human language, and provide responses similar to a human representative. Chatbots can serve customers by answering basic inquiries, walking them through simple tasks, such as resetting a password, or taking account information. Because chatbots are available 24 hours a day, seven days a week, 365 days per year, customers can get their questions answered at their convenience. Over time, chatbots collect data that helps them provide faster, better responses, resulting in positive customer service.
Embracing machine learning
We can expect to see the financial industry continue to embrace machine learning technology. Whether supporting cybersecurity, trading, regulatory technology, detecting fraudulent activity, as well as serving customers, machine learning has the potential to save banks time and money. As banks adopt new technologies into their daily operations, they can use newly freed resources into setting new business standards to better serve their customers.
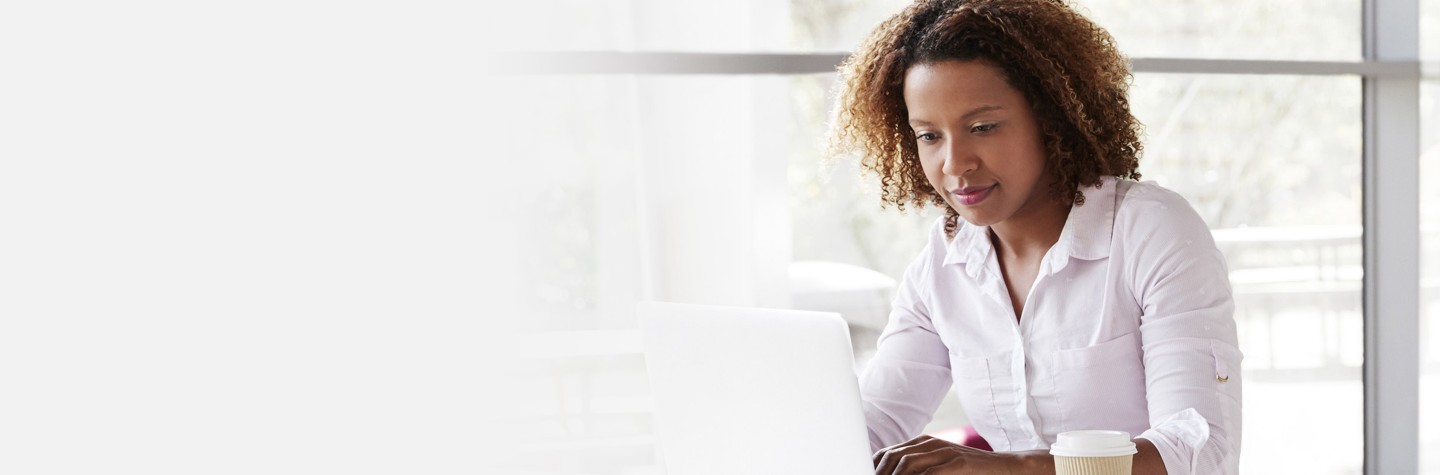
Ready to learn more?
See how Thomson Reuters CLEAR makes it easier to locate people, businesses, assets, and other critical information